Predictive analytics-based evaluation of performance of public bus transportation San Antonio, Texas as a case study
Keywords:
Predictive Analytics, GTFS, Transportation IntelligenceSynopsis
This is a Chapter in:
Book:
Smart and Sustainable Applications
Print ISBN 978-1-6692-0006-2
Online ISBN 978-1-6692-0005-5
Series:
Chronicle of Computing
Chapter Abstract:
Citizens in large cities utilize public transportation as an alternative to self-driving for several reasons, such as avoiding traffic congestion and parking costs and utilizing their time for other things (e.g. reading a book or responding to emails). While large cities provide public transportation as a service to their citizens, they need to consider optimizing their budget and ensuring that public transportation is available and reliable. Using our case study, the public bus transit system in the city of San Antonio, Texas, in this paper, we used predictive analytics models to evaluate the performance of public bus transportation. We used time point stops as the target variable in order to evaluate their impact on the overall performance of the system. We also evaluated methods for the detection of potential bus-time savings and reported several examples of possible savings.
Keywords:
Predictive Analytics, GTFS, Transportation Intelligence
Cite this paper as:
Izzat Alsmadi I., Al-Ramahi M. (2024) Predictive analytics-based evaluation of performance of public bus transportation San Antonio, Texas as a case study. In: Tiako P.F. (ed) Smart and Sustainable Applications. Chronicle of Computing. OkIP. https://doi.org/10.55432/978-1-6692-0005-5_1
Presented at:
The 2023 OkIP International Conference on Automated and Intelligent Systems (CAIS) in Oklahoma City, Oklahoma, USA, and Online, on October 2-5, 2023.
Contact:
Izzat Alsmadi
izzat.alsmadi@tamusa.edu
References
Akse, F. A. (2014). Aggregate waiting time reduction on public transportation networks.
Arias, D., Todd, K., Krieger, J., Maddox, S., Haley, P., Watkins, K. E., & Berrebi, S. (2021). Using gtfs to calculate travel time savings potential of bus preferential treatments. Transportation Research Record, 2675(9), 1643-1654.
Badie Modiri, A. (2018). Error and attack tolerance of public transportation networks: a temporal networks approach.
Butts, k. K. (2021). Retrieved from https://github.com/kuanb/peartree
Danaher, A., Wensley, J., Dunham, A., Orosz, T., Avery, R., Cobb, K., . . . Connor, M. (2020). Minutes Matter: A Bus Transit Service Reliability Guidebook.
Deb, K., Pratap, A., Agarwal, S., & Meyarivan, T. (2002). A fast and elitist multiobjective genetic algorithm: NSGA-II. IEEE transactions on evolutionary computation, 6(2), 182-197.
Delling, D., Pajor, T., & Werneck, R. F. (2015). Round-based public transit routing. Transportation Science, 49(3), 591-604.
Fortin, P., Morency, C., & Trépanier, M. (2016). Innovative GTFS data application for transit network analysis using a graph-oriented method. Journal of Public Transportation, 19(4), 18-37.
Glick, T. B. (2020). Methodologies to Quantify Transit Performance Metrics at the System-Level Using High-Resolution GPS, Stop-Level, and GTFS Archived Transit Data. Portland State University,
Jeon, I., Nam, H., & Jun, C. (2018). A schedule-based public transit routing algorithm for finding K-shortest paths considering transfer penalties. The Journal of The Korea Institute of Intelligent Transport Systems, 17(3), 72-86.
Lee, J., & Miller, H. J. (2020). Robust accessibility: Measuring accessibility based on travelers' heterogeneous strategies for managing travel time uncertainty. Journal of Transport Geography, 86, 102747.
Liu, L., & Miller, H. J. (2020). Does real-time transit information reduce waiting time? An empirical analysis. Transportation Research Part A: Policy and Practice, 141, 167-179.
Madamori, O., Max-Onakpoya, E., Erhardt, G. D., & Baker, C. E. (2021). Enabling opportunistic low-cost smart cities by using tactical edge node placement. Paper presented at the 2021 16th Annual Conference on Wireless On-demand Network Systems and Services Conference (WONS).
Reyna, O. S. S., de la Mota, I. F., & Vázquez, K. R. (2021). Complex networks analysis: Mexico’s city metro system during the pandemic of COVID-19. Case Studies on Transport Policy, 9(4), 1459-1466.
Rothfeld, R., Fu, M., Balać, M., & Antoniou, C. (2021). Potential urban air mobility travel time savings: An exploratory analysis of Munich, Paris, and San Francisco. Sustainability, 13(4), 2217.
Selcraig, B. (2020). Early Success of San Antonio’s VIA Link Prompts Citywide Expansion Plan. Retrieved from https://www.expressnews.com/news/local/article/Early-success-of-San-Antonio-s-VIA-Link-prompts-15075197.php
Sun, F., Dubey, A., White, J., & Gokhale, A. (2019). Transit-hub: A smart public transportation decision support system with multi-timescale analytical services. Cluster Computing, 22, 2239-2254.
Sun, F., Samal, C., White, J., & Dubey, A. (2017). Unsupervised mechanisms for optimizing on-time performance of fixed schedule transit vehicles. Paper presented at the 2017 IEEE International Conference on Smart Computing (SMARTCOMP).
Tesfaye, B., Augsten, N., Pawlik, M., Böhlen, M. H., & Jensen, C. S. (2022). Speeding up reachability queries in public transport networks using graph partitioning. Information Systems Frontiers, 24(1), 11-29.
Zhang, T. (2014). Bus stop usage evaluation and BRT station selection strategy by machine learning methods: State University of New York at Albany.
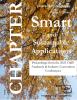